
Genomics workflows, Part 6: cost prediction
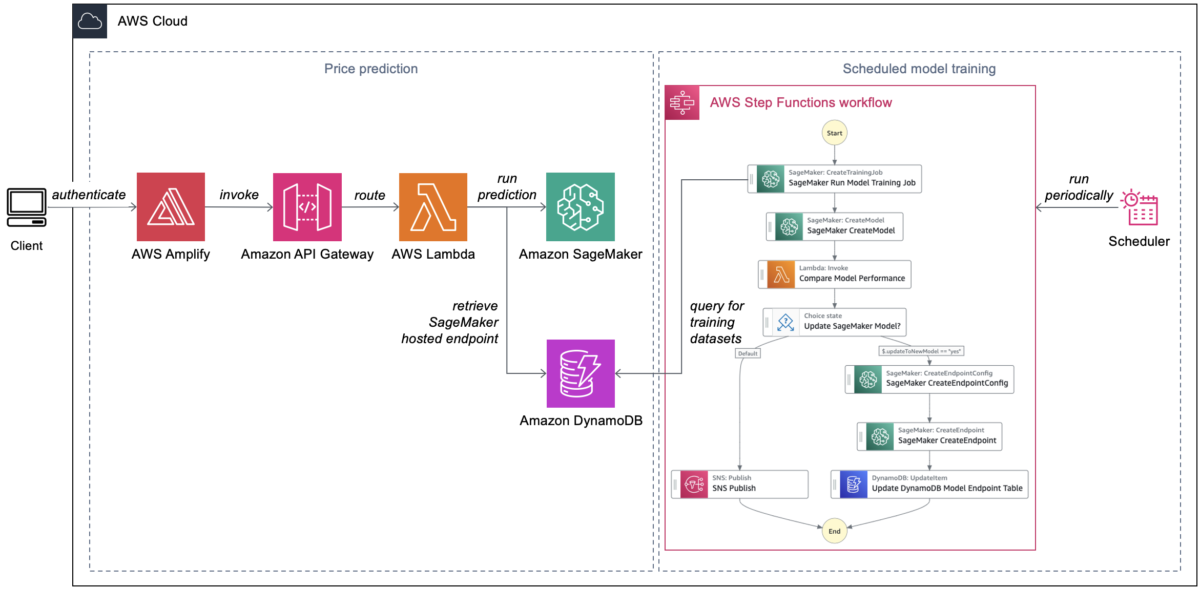
Genomics workflows run on large pools of compute resources and take petabyte-scale datasets as inputs… Given this large scale, scientists want to estimate the projected cost of their genomics workflow runs before deciding to launch them… While we focus on genomics, the design pattern is broadly …
Genomics workflows run on large pools of compute resources and take petabyte-scale datasets as inputs. Workflow runs can cost as much as hundreds of thousands of US dollars. Given this large scale, scientists want to estimate the projected cost of their genomics workflow runs before deciding to launch them.
In Part 6 of this series, we build on the benchmarking concepts presented in Part 5. You will learn how to train machine learning (ML) models on historical data to predict the cost of future runs. While we focus on genomics, the design pattern is broadly applicable to any compute-intensive workflow use case.
Use case
In large life-sciences organizations, multiple research teams often use the same genomics applications. The actual cost of consuming shared resources is only periodically shown or charged back to research teams.
In this blog post’s scenario, scientists want to predict the cost of future workflow runs based on the following input parameters:
- Workflow name
- Input data set size
- Expected output dataset size
In our experience, scientists might not know how to reliably estimate compute cost based on the preceding parameters. This is because workflow run cost doesn’t linearly correlate to the input dataset size. For example, some workflow steps might be highly parallelizable while others aren’t. Otherwise, scientists could simply use the AWS Pricing Calculator or interact programmatically with the AWS Price List API. To solve this problem, we use ML to model the pattern of correlation and predict workflow cost.
Business benefits of predicting the cost of genomics workflow runs
Price prediction brings the following benefits:
- Prioritizing workflow runs based on financial impact
- Promoting cost awareness and frugality with application users
- Supporting enterprise resource planning and prevention of budget overruns by integrating estimation data into management reporting and approval workflows
Prerequisites
To build this solution, you must have workflows running on AWS for which you collect actual cost data after each workflow run. This setup is demonstrated in Part 3 and Part 5 of this blog series. This data provides training data for the solution’s cost prediction models.
Solution overview
This solution includes a friendly user interface, ML models that predict usage parameters, and a metadata storage mechanism to estimate the cost of a workflow run. We use the automated workflow manager presented in Part 3 and the benchmarking solution from Part 5. The data on historical workflow launches and their cost serves as training and testing data for our ML models. We store this in Amazon DynamoDB. We use AWS Amplify to host a serverless user interface and a library/framework such as React to build it.
Scientists input the required parameters about their genomics workflow run to the Amplify frontend React application. The latter makes a request to an Amazon API Gateway REST API. This invokes an AWS Lambda function, which calls an Amazon SageMaker hosted endpoint to return predicted costs (Figure 1).
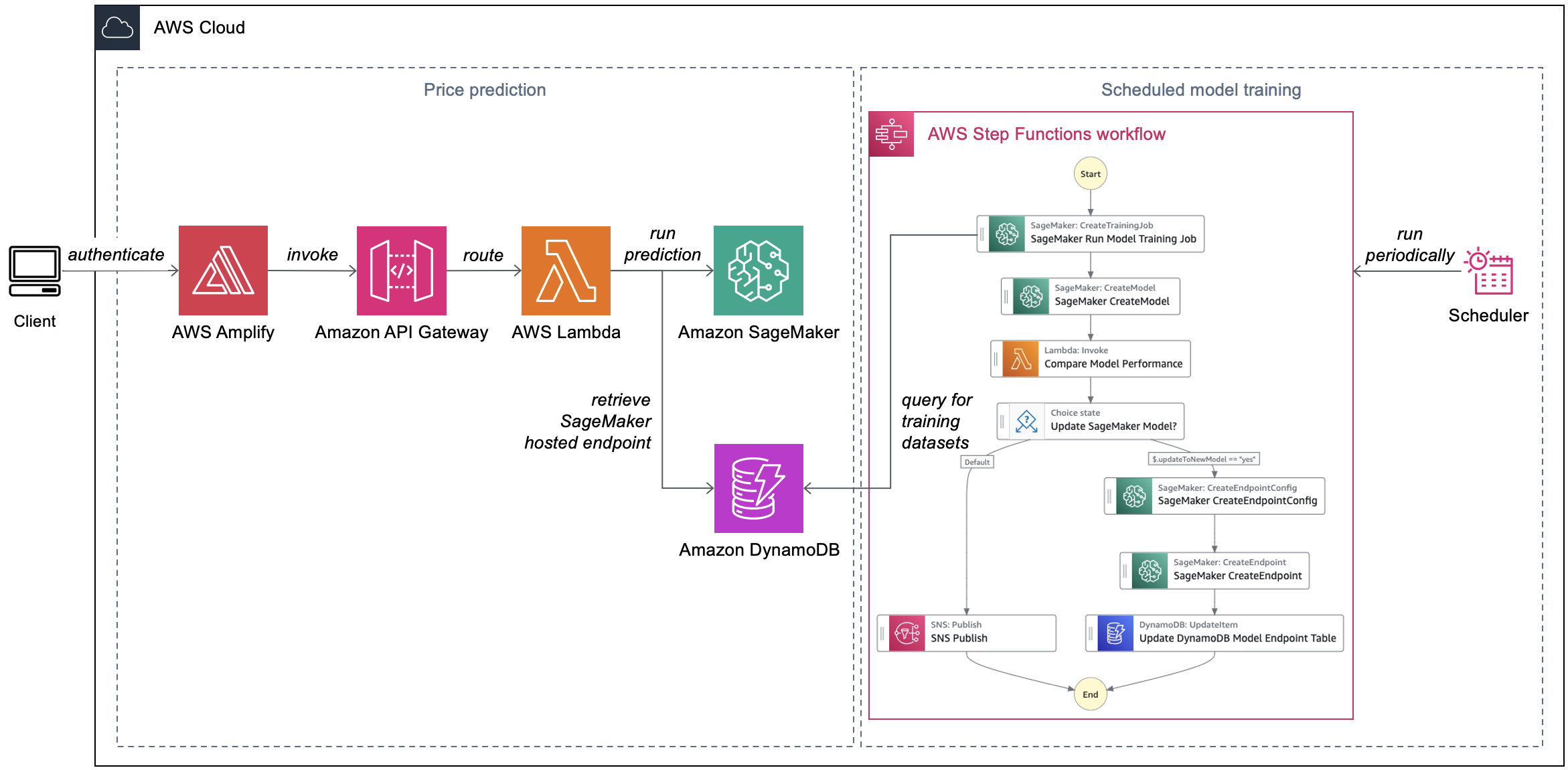
Figure 1. Automated cost prediction of genomics workflow runs
Each workflow captured in the DynamoDB table has a corresponding ML model trained for the specific use case. Separating out models for specific workflows simplifies the model development process. This solution periodically trains ML models to improve their overall accuracy and performance. A rule in Amazon EventBridge Scheduler invokes model training on a regular basis. An AWS Step Functions state machine automates the model training process.
Implementation considerations
When a scientist submits a request (which includes the name of the workflow they’re running), API Gateway uses Lambda integration. The Lambda function retrieves a record from the DynamoDB table that keeps track of the SageMaker hosted endpoints. The partition key of the table is the workflow name (indicated as workflow_name
), as shown in the following example:
Using the input parameters, the Lambda function invokes the SageMaker hosted endpoint and returns the inference values back to the frontend.
Automating model training
Our Step Functions state machine for model training uses native SageMaker SDK integration. It runs as follows:
- The state machine invokes a SageMaker training job to train a new ML model. The training job uses the historical workflow run data sourced from the DynamoDB table. After the training job completes, it outputs the ML model to an Amazon Simple Storage Service (Amazon S3) bucket.
- The state machine registers the new model in the SageMaker model registry.
- A Lambda function compares the performance of the new model with the prior version on the training dataset.
- If the new model performs better than the prior model, the state machine creates a new SageMaker hosted endpoint configuration and puts the endpoint name in the DynamoDB table.
- Otherwise, the state machine sends a notification to an Amazon Simple Notification Service (Amazon SNS) topic stating that there are no updates.
Conclusion
In this blog post, we demonstrated how genomics research teams can build a price estimator to predict genomics workflow run cost. This solution trains ML models for each workflow based on data from historical workflow runs. A state machine helps automate the entire model training process. You can use price estimation to promote cost awareness in your organization and reduce the risk of budget overruns.
Our solution is particularly suitable if you want to predict the price of individual workflow runs. If you want forecast overall consumption of your shared application infrastructure, consider deploying a forecasting workflow with Amazon Forecast. The Build workflows for Amazon Forecast with AWS Step Functions blog post provides details on the specific use case for using Amazon Forecast workflows.
Related information
- Genomics workflows, Part 1: automated launches
- Genomics workflows, Part 2: simplify Snakemake launches
- Genomics workflows, Part 3: automated workflow manager
- Genomics workflows, Part 4: processing archival data
- Genomics workflows, Part 5: automated benchmarking
- Build workflows for Amazon Forecast with AWS Step Functions
- Genomics on AWS
- Amazon Genomics CLI
Author: Rostislav Markov