
From RAG to fabric: Lessons learned from building real-world RAGs at GenAIIC – Part 1
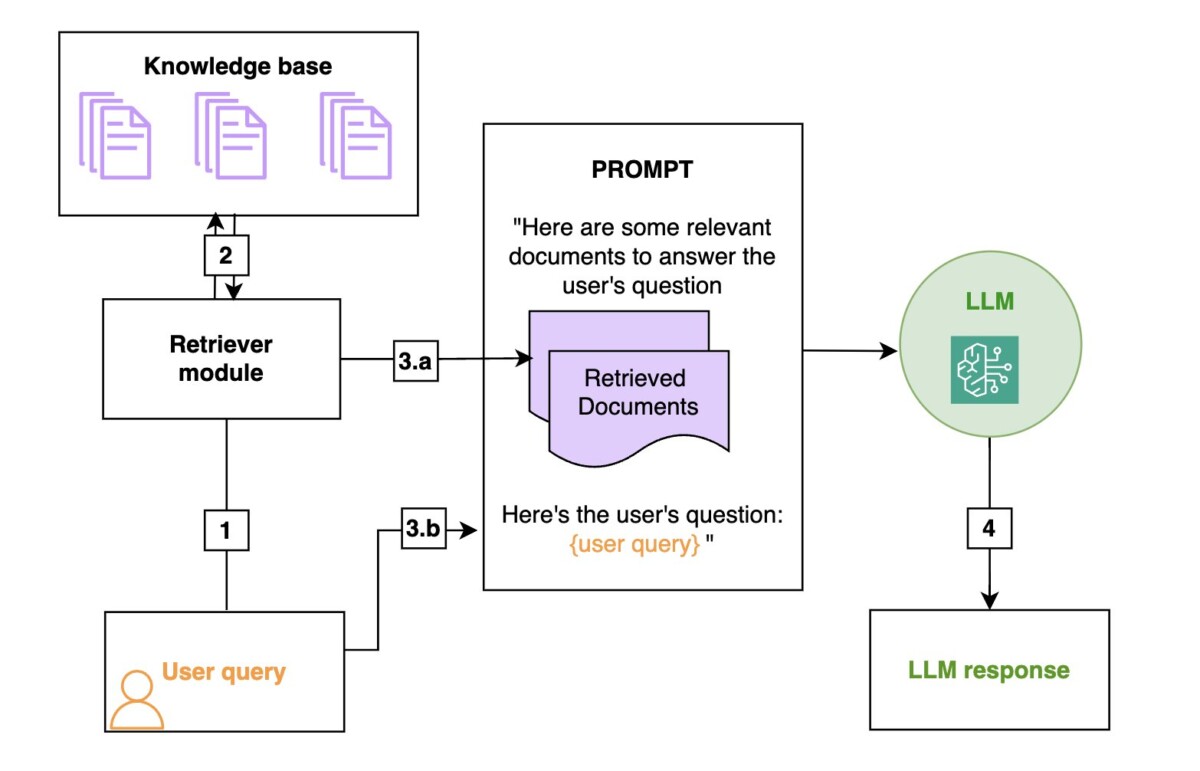
But what is a retriever exactly? Broadly speaking, a retriever is a module that takes a query as input and outputs relevant documents from one or more knowledge sources relevant to that query… Document ingestion In a RAG architecture, documents are often stored in a vector store… As shown in…
The AWS Generative AI Innovation Center (GenAIIC) is a team of AWS science and strategy experts who have deep knowledge of generative AI. They help AWS customers jumpstart their generative AI journey by building proofs of concept that use generative AI to bring business value. Since the inception of AWS GenAIIC in May 2023, we have witnessed high customer demand for chatbots that can extract information and generate insights from massive and often heterogeneous knowledge bases. Such use cases, which augment a large language model’s (LLM) knowledge with external data sources, are known as Retrieval-Augmented Generation (RAG).
This two-part series shares the insights gained by AWS GenAIIC from direct experience building RAG solutions across a wide range of industries. You can use this as a practical guide to building better RAG solutions.
In this first post, we focus on the basics of RAG architecture and how to optimize text-only RAG. The second post outlines how to work with multiple data formats such as structured data (tables, databases) and images.
Anatomy of RAG
RAG is an efficient way to provide an FM with additional knowledge by using external data sources and is depicted in the following diagram:
- Retrieval: Based on a user’s question (1), relevant information is retrieved from a knowledge base (2) (for example, an OpenSearch index).
- Augmentation: The retrieved information is added to the FM prompt (3.a) to augment its knowledge, along with the user query (3.b).
- Generation: The FM generates an answer (4) by using the information provided in the prompt.
The following is a general diagram of a RAG workflow. From left to right are the retrieval, the augmentation, and the generation. In practice, the knowledge base is often a vector store.
A deeper dive in the retriever
In a RAG architecture, the FM will base its answer on the information provided by the retriever. Therefore, a RAG is only as good as its retriever, and many of the tips that we share in our practical guide are about how to optimize the retriever. But what is a retriever exactly? Broadly speaking, a retriever is a module that takes a query as input and outputs relevant documents from one or more knowledge sources relevant to that query.
Document ingestion
In a RAG architecture, documents are often stored in a vector store. As shown in the following diagram, vector stores are populated by chunking the documents into manageable pieces (1) (if a document is short enough, chunking might not be required) and transforming each chunk of the document into a high-dimensional vector using a vector embedding (2), such as the Amazon Titan embeddings model. These embeddings have the characteristic that two chunks of texts that are semantically close have vector representations that are also close in that embedding (in the sense of the cosine or Euclidean distance).
The following diagram illustrates the ingestion of text documents in the vector store using an embedding model. Note that the vectors are stored alongside the corresponding text chunk (3), so that at retrieval time, when you identify the chunks closest to the query, you can return the text chunk to be passed to the FM prompt.
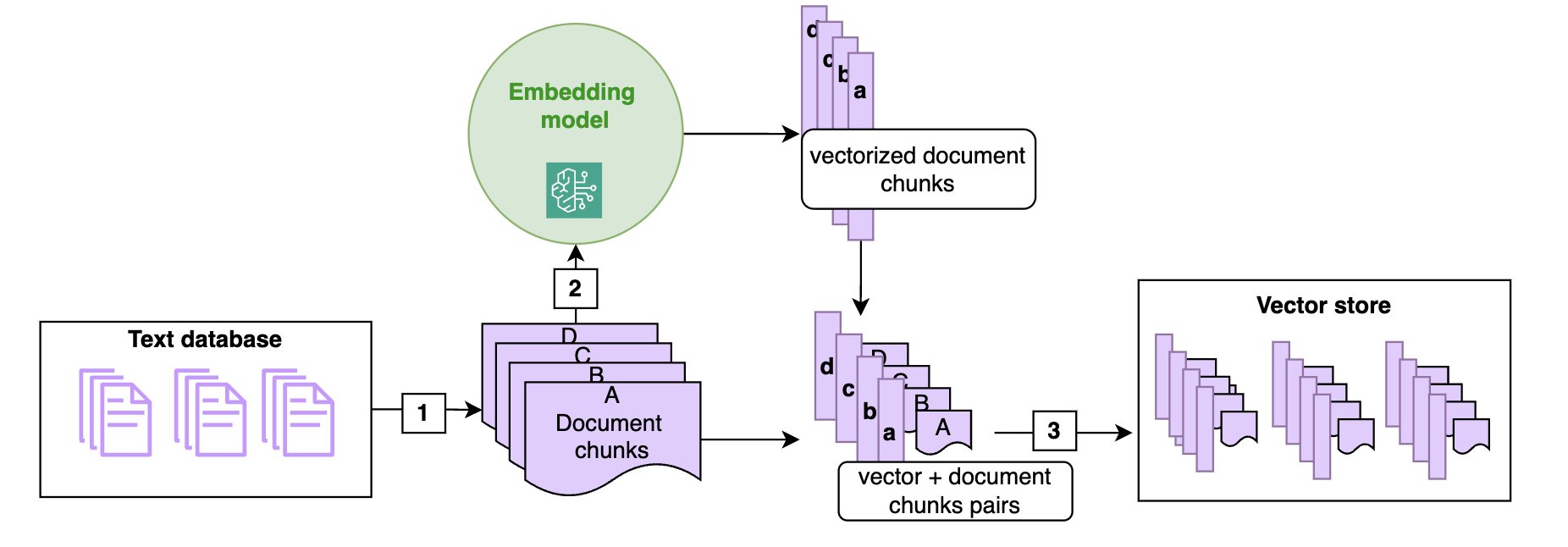
Semantic search
Vector stores allow for efficient semantic search: as shown in the following diagram, given a user query (1), we vectorize it (2) (using the same embedding as the one that was used to build the vector store) and then look for the nearest vectors in the vector store (3), which will correspond to the document chunks that are semantically closest to the initial query (4). Although vector stores and semantic search have become the default in RAG architectures, more traditional keyword-based search is still valuable, especially when searching for domain-specific words (such as technical jargon) or names. Hybrid search is a way to use both semantic search and keywords to rank a document, and we will give more details on this technique in the section on advanced RAG techniques.
The following diagram illustrates the retrieval of text documents that are semantically close to the user query. You must use the same embedding model at ingestion time and at search time.
Implementation on AWS
A RAG chatbot can be set up in a matter of minutes using Amazon Bedrock Knowledge Bases. The knowledge base can be linked to an Amazon Simple Storage Service (Amazon S3) bucket and will automatically chunk and index the documents it contains in an OpenSearch index, which will act as the vector store. The retrieve_and_generate
API does both the retrieval and a call to an FM (Amazon Titan or Anthropic’s Claude family of models on Amazon Bedrock), for a fully managed solution. The retrieve API only implements the retrieval component and allows for a more custom approach downstream, such as document post processing before calling the FM separately.
In this blog post, we will provide tips and code to optimize a fully custom RAG solution with the following components:
- An OpenSearch Serverless vector search collection as the vector store
- Custom chunking and ingestion functions to ingest the documents in the OpenSearch index
- A custom retrieval function that takes a user query as an input and outputs the relevant documents from the OpenSearch index
- FM calls to your model of choice on Amazon Bedrock to generate the final answer.
In this post, we focus on a custom solution to help readers understand the inner workings of RAG. Most of the tips we provide can be adapted to work with Amazon Bedrock Knowledge Bases, and we will point this out in the relevant sections.
Overview of RAG use cases
While working with customers on their generative AI journey, we encountered a variety of use cases that fit within the RAG paradigm. In traditional RAG use cases, the chatbot relies on a database of text documents (.doc, .pdf, or .txt). In part 2 of this post, we will discuss how to extend this capability to images and structured data. For now, we’ll focus on a typical RAG workflow: the input is a user question, and the output is the answer to that question, derived from the relevant text chunks or documents retrieved from the database. Use cases include the following:
- Customer service– This can include the following:
- Internal– Live agents use an internal chatbot to help them answer customer questions.
- External– Customers directly chat with a generative AI chatbot.
- Hybrid– The model generates smart replies for live agents that they can edit before sending to customers.
- Employee training and resources– In this use case, chatbots can use employee training manuals, HR resources, and IT service documents to help employees onboard faster or find the information they need to troubleshoot internal issues.
- Industrial maintenance– Maintenance manuals for complex machines can have several hundred pages. Building a RAG solution around these manuals helps maintenance technicians find relevant information faster. Note that maintenance manuals often have images and schemas, which could put them in a multimodal bucket.
- Product information search– Field specialists need to identify relevant products for a given use case, or conversely find the right technical information about a given product.
- Retrieving and summarizing financial news– Analysts need the most up-to-date information on markets and the economy and rely on large databases of news or commentary articles. A RAG solution is a way to efficiently retrieve and summarize the relevant information on a given topic.
In the following sections, we will give tips that you can use to optimize each aspect of the RAG pipeline (ingestion, retrieval, and answer generation) depending on the underlying use case and data format. To verify that the modifications improve the solution, you first need to be able to assess the performance of the RAG solution.
Evaluating a RAG solution
Contrary to traditional machine learning (ML) models, for which evaluation metrics are well defined and straightforward to compute, evaluating a RAG framework is still an open problem. First, collecting ground truth (information known to be correct) for the retrieval component and the generation component is time consuming and requires human intervention. Secondly, even with several question-and-answer pairs available, it’s difficult to automatically evaluate if the RAG answer is close enough to the human answer.
In our experience, when a RAG system performs poorly, we found the retrieval part to almost always be the culprit. Large pre-trained models such as Anthropic’s Claude model will generate high-quality answers if provided with the right information, and we notice two main failure modes:
- The relevant information isn’t present in the retrieved documents: In this case, the FM can try to make up an answer or use its own knowledge to answer. Adding guardrails against such behavior is essential.
- Relevant information is buried within an excessive amount of irrelevant data: When the scope of the retriever is too broad, the FM can get confused and start mixing up multiple data sources, resulting in a wrong answer. More advanced models such as Anthropic’s Claude Sonnet 3.5 and Opus are reported to be more robust against such behavior, but this is still a risk to be aware of.
To evaluate the quality of the retriever, you can use the following traditional retrieval metrics:
- Top-k accuracy: Measures whether at least one relevant document is found within the top k retrieved documents.
- Mean Reciprocal Rank (MRR)– This metric considers the ranking of the retrieved documents. It’s calculated as the average of the reciprocal ranks (RR) for each query. The RR is the inverse of the rank position of the first relevant document. For example, if the first relevant document is in third position, the RR is 1/3. A higher MRR indicates that the retriever can rank the most relevant documents higher.
- Recall– This metric measures the ability of the retriever to retrieve relevant documents from the corpus. It’s calculated as the number of relevant documents that are successfully retrieved over the total number of relevant documents. Higher recall indicates that the retriever can find most of the relevant information.
- Precision– This metric measures the ability of the retriever to retrieve only relevant documents and avoid irrelevant ones. It’s calculated by the number of relevant documents successfully retrieved over the total number of documents retrieved. Higher precision indicates that the retriever isn’t retrieving too many irrelevant documents.
Note that if the documents are chunked, the metrics must be computed at the chunk level. This means the ground truth to evaluate a retriever is pairs of question and list of relevant document chunks. In many cases, there is only one chunk that contains the answer to the question, so the ground truth becomes question and relevant document chunk.
To evaluate the quality of the generated response, two main options are:
- Evaluation by subject matter experts: this provides the highest reliability in terms of evaluation but can’t scale to a large number of questions and slows down iterations on the RAG solution.
- Evaluation by FM (also called LLM-as-a-judge):
- With a human-created starting point: Provide the FM with a set of ground truth question-and-answer pairs and ask the FM to evaluate the quality of the generated answer by comparing it to the ground truth one.
- With an FM-generated ground truth: Use an FM to generate question-and-answer pairs for given chunks, and then use this as a ground truth, before resorting to an FM to compare RAG answers to that ground truth.
We recommend that you use an FM for evaluations to iterate faster on improving the RAG solution, but to use subject-matter experts (or at least human evaluation) to provide a final assessment of the generated answers before deploying the solution.
A growing number of libraries offer automated evaluation frameworks that rely on additional FMs to create a ground truth and evaluate the relevance of the retrieved documents as well as the quality of the response:
- Ragas– This framework offers FM-based metrics previously described, such as context recall, context precision, answer faithfulness, and answer relevancy. It needs to be adapted to Anthropic’s Claude models because of its heavy dependence on specific prompts.
- LlamaIndex– This framework provides multiple modules to independently evaluate the retrieval and generation components of a RAG system. It also integrates with other tools such as Ragas and DeepEval. It contains modules to create ground truth (query-and-context pairs and question-and-answer pairs) using an FM, which alleviates the use of time-consuming human collection of ground truth.
- RefChecker– This is an Amazon Science library focused on fine-grained hallucination detection.
Troubleshooting RAG
Evaluation metrics give an overall picture of the performance of retrieval and generation, but they don’t help diagnose issues. Diving deeper into poor responses can help you understand what’s causing them and what you can do to alleviate the issue. You can diagnose the issue by looking at evaluation metrics and also by having a human evaluator take a closer look at both the LLM answer and the retrieved documents.
The following is a brief overview of issues and potential fixes. We will describe each of the techniques in more detail, including real-world use cases and code examples, in the next section.
- The relevant chunk wasn’t retrieved (retriever has low top k accuracy and low recall or spotted by human evaluation):
- Try increasing the number of documents retrieved by the nearest neighbor search and re-ranking the results to cut back on the number of chunks after retrieval.
- Try hybrid search. Using keywords in combination with semantic search (known as hybrid search) might help, especially if the queries contain names or domain-specific jargon.
- Try query rewriting. Having an FM detect the intent or rewrite the query can help create a query that’s better suited for the retriever. For instance, a user query such as “What information do you have in the knowledge base about the economic outlook in China?” contains a lot of context that isn’t relevant to the search and would be more efficient if rewritten as “economic outlook in China” for search purposes.
- Too many chunks were retrieved (retriever has low precision or spotted by human evaluation):
- Try using keyword matching to restrict the search results. For example, if you’re looking for information about a specific entity or property in your knowledge base, only retrieve documents that explicitly mention them.
- Try metadata filtering in your OpenSearch index. For example, if you’re looking for information in news articles, try using the date field to filter only the most recent results.
- Try using query rewriting to get the right metadata filtering. This advanced technique uses the FM to rewrite the user query as a more structured query, allowing you to make the most of OpenSearch filters. For example, if you’re looking for the specifications of a specific product in your database, the FM can extract the product name from the query, and you can then use the product name field to filter out the product name.
- Try using reranking to cut down on the number of chunks passed to the FM.
- A relevant chunk was retrieved, but it’s missing some context (can only be assessed by human evaluation):
- Try changing the chunking strategy. Keep in mind that small chunks are good for precise questions, while large chunks are better for questions that require a broad context:
- Try increasing the chunk size and overlap as a first step.
- Try using section-based chunking. If you have structured documents, use sections delimiters to cut your documents into chunks to have more coherent chunks. Be aware that you might lose some of the more fine-grained context if your chunks are larger.
- Try small-to-large retrievers. If you want to keep the fine-grained details of small chunks but make sure you retrieve all the relevant context, small-to-large retrievers will retrieve your chunk along with the previous and next ones.
- Try changing the chunking strategy. Keep in mind that small chunks are good for precise questions, while large chunks are better for questions that require a broad context:
- If none of the above help:
- Consider training a custom embedding.
- The retriever isn’t at fault, the problem is with FM generation (evaluated by a human or LLM):
- Try prompt engineering to mitigate hallucinations.
- Try prompting the FM to use quotes in its answers, to allow for manual fact checking.
- Try using another FM to evaluate or correct the answer.
A practical guide to improving the retriever
Note that not all the techniques that follow need to be implemented together to optimize your retriever—some might even have opposite effects. Use the preceding troubleshooting guide to get a shortlist of what might work, then look at the examples in the corresponding sections that follow to assess if the method can be beneficial to your retriever.
Hybrid search
Example use case: A large manufacturer built a RAG chatbot to retrieve product specifications. These documents contain technical terms and product names. Consider the following example queries:
The queries are equivalent and need to be answered with the same document. The keyword component will make sure that you’re boosting documents mentioning the name of the product, XYZ
while the semantic component will make sure that documents containing viscosity
get a high score, even when the query contains the word viscous
.
Combining vector search with keyword search can effectively handle domain-specific terms, abbreviations, and product names that embedding models might struggle with. Practically, this can be achieved in OpenSearch by combining a k-nearest neighbors (k-NN) query with keyword matching. The weights for the semantic search compared to keyword search can be adjusted. See the following example code:
Amazon Bedrock Knowledge Bases also supports hybrid search, but you can’t adjust the weights for semantic compared to keyword search.
Adding metadata information to text chunks
Example use case: Using the same example of a RAG chatbot for product specifications, consider product specifications that are several pages long and where the product name is only present in the header of the document. When ingesting the document into the knowledge base, it’s chunked into smaller pieces for the embedding model, and the product name only appears in the first chunk, which contains the header. See the following example:
Below is the FM response to the question "What are the strengths of player A?"
:
Author: Aude Genevay