
Meta Llama 3 models are now available in Amazon SageMaker JumpStart
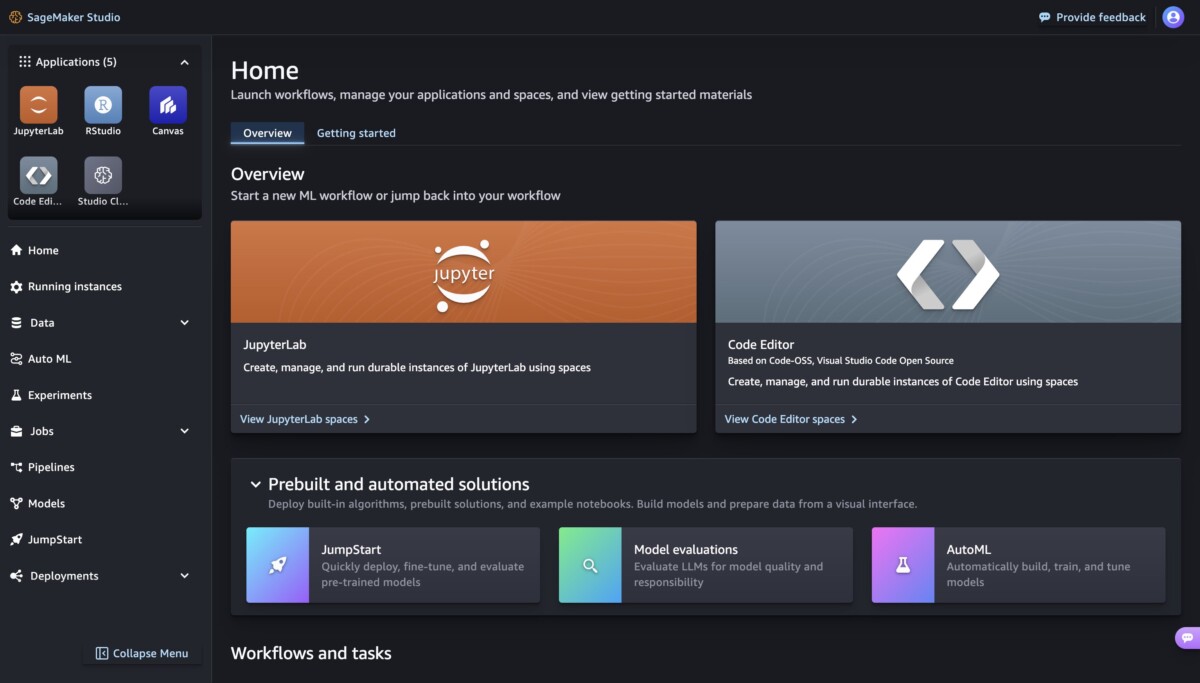
Today, we are excited to announce that Meta Llama 3 foundation models are available through Amazon SageMaker JumpStart to deploy and run inference… The Llama 3 models are a collection of pre-trained and fine-tuned generative text models… In this post, we walk through how to discover and deploy…
Today, we are excited to announce that Meta Llama 3 foundation models are available through Amazon SageMaker JumpStart to deploy and run inference. The Llama 3 models are a collection of pre-trained and fine-tuned generative text models.
In this post, we walk through how to discover and deploy Llama 3 models via SageMaker JumpStart.
What is Meta Llama 3
Llama 3 comes in two parameter sizes — 8B and 70B with 8k context length — that can support a broad range of use cases with improvements in reasoning, code generation, and instruction following. Llama 3 uses a decoder-only transformer architecture and new tokenizer that provides improved model performance with 128k size. In addition, Meta improved post-training procedures that substantially reduced false refusal rates, improved alignment, and increased diversity in model responses. You can now derive the combined advantages of Llama 3 performance and MLOps controls with Amazon SageMaker features such as SageMaker Pipelines, SageMaker Debugger, or container logs. In addition, the model will be deployed in an AWS secure environment under your VPC controls, helping provide data security.
What is SageMaker JumpStart
With SageMaker JumpStart, you can choose from a broad selection of publicly available foundation models. ML practitioners can deploy foundation models to dedicated SageMaker instances from a network isolated environment and customize models using SageMaker for model training and deployment. You can now discover and deploy Llama 3 models with a few clicks in Amazon SageMaker Studio or programmatically through the SageMaker Python SDK, enabling you to derive model performance and MLOps controls with SageMaker features such as SageMaker Pipelines, SageMaker Debugger, or container logs. The model is deployed in an AWS secure environment and under your VPC controls, helping provide data security. Llama 3 models are available today for deployment and inferencing in Amazon SageMaker Studio in us-east-1
(N. Virginia), us-east-2
(Ohio), us-west-2
(Oregon), eu-west-1
(Ireland) and ap-northeast-1
(Tokyo) AWS Regions.
Discover models
You can access the foundation models through SageMaker JumpStart in the SageMaker Studio UI and the SageMaker Python SDK. In this section, we go over how to discover the models in SageMaker Studio.
SageMaker Studio is an integrated development environment (IDE) that provides a single web-based visual interface where you can access purpose-built tools to perform all ML development steps, from preparing data to building, training, and deploying your ML models. For more details on how to get started and set up SageMaker Studio, refer to Amazon SageMaker Studio.
In SageMaker Studio, you can access SageMaker JumpStart, which contains pre-trained models, notebooks, and prebuilt solutions, under Prebuilt and automated solutions.
From the SageMaker JumpStart landing page, you can easily discover various models by browsing through different hubs which are named after model providers. You can find Llama 3 models in Meta hub. If you do not see Llama 3 models, please update your SageMaker Studio version by shutting down and restarting. For more information, refer to Shut down and Update Studio Classic Apps.
You can find Llama 3 models by searching for “Meta-llama-3“ from the search box located at top left.
You can discover all Meta models available in SageMaker JumpStart by clicking on Meta hub.
Clicking on a model card opens the corresponding model detail page, from which you can easily Deploy the model.
Deploy a model
When you choose Deploy and acknowledge the EULA terms, deployment will start.
You can monitor progress of the deployment on the page that shows up after clicking the Deploy button.
Alternatively, you can choose Open notebook to deploy through the example notebook. The example notebook provides end-to-end guidance on how to deploy the model for inference and clean up resources.
To deploy using the notebook, you start by selecting an appropriate model, specified by the model_id
. You can deploy any of the selected models on SageMaker with the following code.
By default accept_eula
is set to False
. You need to manually accept the EULA to deploy the endpoint successfully, By doing so, you accept the user license agreement and acceptable use policy. You can also find the license agreement Llama website. This deploys the model on SageMaker with default configurations including the default instance type and default VPC configurations. You can change these configuration by specifying non-default values in JumpStartModel
. To learn more, please refer to the following documentation.
The following table lists all the Llama 3 models available in SageMaker JumpStart along with the model_ids
, default instance types and maximum number of total tokens (sum of the number of input tokens and number of generated tokens) supported for each of these models.
Model Name | Model ID | Max Total Tokens | Default instance type |
Meta-Llama-3-8B | meta-textgeneration-llama-3-8B | 8192 | ml.g5.12xlarge |
Meta-Llama-3-8B-Instruct | meta-textgeneration-llama-3-8B-instruct | 8192 | ml.g5.12xlarge |
Meta-Llama-3-70B | meta-textgeneration-llama-3-70b | 8192 | ml.p4d.24xlarge |
Meta-Llama-3-70B-Instruct | meta-textgeneration-llama-3-70b-instruct | 8192 | ml.p4d.24xlarge |
Run inference
After you deploy the model, you can run inference against the deployed endpoint through SageMaker predictor. Fine-tuned instruct models (Llama 3: 8B Instruct and 70B Instruct) accept a history of chats between the user and the chat assistant, and generate the subsequent chat. The pre-trained models (Llama 3: 8B and 70B) require a string prompt and perform text completion on the provided prompt.
Inference parameters control the text generation process at the endpoint. The Max new tokens control the size of the output generated by the model. This is not same as the number of words because the vocabulary of the model is not the same as the English language vocabulary, and each token may not be an English language word. The temperature parameter controls the randomness in the output. Higher temperature results in more creative and hallucinated outputs. All the inference parameters are optional.
Example prompts for the 70B model
You can use Llama 3 models for text completion for any piece of text. Through text generation, you can perform a variety of tasks such as question answering, language translation, and sentiment analysis, and more. The input payload to the endpoint looks like the following code:
The following are some sample example prompts and the text generated by the model. All outputs are generated with inference parameters {"max_new_tokens":64, "top_p":0.9, "temperature":0.6}
.
In the next example, we show how to use Llama 3 models with few shot in-context learning where we provide training samples available to the model. We only run inference on the deployed model and during this process, and model weights do not change.
Example prompts for the 70B-Instruct model
With Llama 3 instruct models which are optimized for dialogue use cases, the input to the instruct model endpoints is the previous history between the chat assistant and the user. You can ask questions contextual to the conversation that has happened so far. You can also provide the system configuration, such as personas, which define the chat assistant’s behavior. While the input payload format is the same as the base pre-trained model, the input text should be formatted in the following manner:
In this instruction template, you can optionally start with a system
role and include as many alternating roles as desired in the turn-based history. The final role should always be assistant
and end with two new line feeds.
Next, consider a few example prompts and responses from the model. In the following example, the user is asking a simple question to the assistant.
In the following example, the user has a conversation with the assistant about tourist sites in Paris. Then the user inquires about the first option recommended by the chat assistant.
In the following examples, we set the system’s configuration.
Clean up
After you’re done running the notebook, make sure to delete all the resources that you created in the process so your billing is stopped. Use the following code:
Conclusion
In this post, we showed you how to get started with Llama 3 models in SageMaker Studio. You now have access to four Llama 3 foundation models that contain billions of parameters. Because foundation models are pretrained, they can also help lower training and infrastructure costs and enable customization for your use case. Check out SageMaker JumpStart in SageMaker Studio now to get started.
- SageMaker JumpStart documentation
- SageMaker JumpStart Foundation Models documentation
- SageMaker JumpStart product detail page
- SageMaker JumpStart model catalog
About Authors
Kyle Ulrich is an Applied Scientist II at AWS
Xin Huang is a Senior Applied Scientist at AWS
Qing Lan is a Senior Software Developer Engineer at AWS
Haotian An is a Software Developer Engineer II at AWS
Christopher Whitten is a Software Development Engineer II at AWS
Tyler Osterberg is a Software Development Engineer I at AWS
Manan Shah is a Software Development Manager at AWS
Jonathan Guinegagne is a Senior Software Developer Engineer at AWS
Adriana Simmons is a Senior Product Marketing Manager at AWS
June Won is a Senior Product Manager at AWS
Ashish Khetan is a Senior Applied Scientist at AWS
Rachna Chadha is a Principal Solution Architect – AI/ML at AWS
Deepak Rupakula is a Principal GTM Specialist at AWS
Author: Kyle Ulrich