
Perform batch transforms with Amazon SageMaker Jumpstart Text2Text Generation large language models
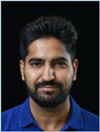
Today we are excited to announce that you can now perform batch transforms with Amazon SageMaker JumpStart large language models (LLMs) for Text2Text Generation… For batch transform, a batch job is run that takes batch input as a dataset and a pre-trained model, and outputs predictions for each da…
Today we are excited to announce that you can now perform batch transforms with Amazon SageMaker JumpStart large language models (LLMs) for Text2Text Generation. Batch transforms are useful in situations where the responses don’t need to be real time and therefore you can do inference in batch for large datasets in bulk. For batch transform, a batch job is run that takes batch input as a dataset and a pre-trained model, and outputs predictions for each data point in the dataset. Batch transform is cost-effective because unlike real-time hosted endpoints that have persistent hardware, batch transform clusters are torn down when the job is complete and therefore the hardware is only used for the duration of the batch job.
In some use cases, real-time inference requests can be grouped in small batches for batch processing to create real-time or near-real-time responses. For example, if you need to process a continuous stream of data with low latency and high throughput, invoking a real-time endpoint for each request separately would require more resources and can take longer to process all the requests because the processing is being done serially. A better approach would be to group some of the requests and call the real-time endpoint in batch inference mode, which processes your requests in one forward pass of the model and returns the bulk response for the request in real time or near-real time. The latency of the response will depend upon how many requests you group together and instance memory size, therefore you can tune the batch size per your business requirements for latency and throughput. We call this real-time batch inference because it combines the concept of batching while still providing real-time responses. With real-time batch inference, you can achieve a balance between low latency and high throughput, enabling you to process large volumes of data in a timely and efficient manner.
Jumpstart batch transform for Text2Text Generation models allows you to pass the batch hyperparameters through environment variables that further increase throughput and minimize latency.
JumpStart provides pretrained, open-source models for a wide range of problem types to help you get started with machine learning (ML). You can incrementally train and tune these models before deployment. JumpStart also provides solution templates that set up infrastructure for common use cases, and executable example notebooks for ML with Amazon SageMaker. You can access the pre-trained models, solution templates, and examples through the JumpStart landing page in Amazon SageMaker Studio. You can also access JumpStart models using the SageMaker Python SDK.
In this post, we demonstrate how to use the state-of-the-art pre-trained text2text FLAN T5 models from Hugging Face for batch transform and real-time batch inference.
Solution overview
The notebook showing batch transform of pre-trained Text2Text FLAN T5 models from Hugging Face in available in the following GitHub repository. This notebook uses data from the Hugging Face cnn_dailymail dataset for a text summarization task using the SageMaker SDK.
The following are the key steps for implementing batch transform and real-time batch inference:
- Set up prerequisites.
- Select a pre-trained model.
- Retrieve artifacts for the model.
- Specify batch transform job hyperparameters.
- Prepare data for the batch transform.
- Run the batch transform job.
- Evaluate the summarization using a ROUGE (Recall-Oriented Understudy for Gisting Evaluation) score.
- Perform real-time batch inference.
Set up prerequisites
Before you run the notebook, you must complete some initial setup steps. Let’s set up the SageMaker execution role so it has permissions to run AWS services on your behalf:
Select a pre-trained model
We use the huggingface-text2text-flan-t5-large model as a default model. Optionally, you can retrieve the list of available Text2Text models on JumpStart and choose your preferred model. This method provides a straightforward way to select different model IDs using same notebook. For demonstration purposes, we use the huggingface-text2text-flan-t5-large model:
Retrieve artifacts for the model
With SageMaker, we can perform inference on the pre-trained model, even without fine-tuning it first on a new dataset. We start by retrieving the deploy_image_uri
, deploy_source_uri
, and model_uri
for the pre-trained model:
Specify batch transform job hyperparameters
You may pass any subset of hyperparameters as environment variables to the batch transform job. You can also pass these hyperparameters in a JSON payload. However, if you’re setting environment variables for hyperparameters like the following code shows, then the advanced hyperparameters from the individual examples in the JSON lines payload will not be used. If you want to use hyperparameters from the payload, you may want to set the hyper_params_dict
parameter as null instead.
Prepare data for batch transform
Now we’re ready to load the cnn_dailymail dataset from Hugging Face:
We go over each data entry and create the input data in the required format. We create an articles.jsonl
file as a test data file containing articles that need to be summarized as input payload. As we create this file, we append the prompt "Briefly summarize this text:"
to each test input row. If you want to have different hyperparameters for each test input, you can append those hyperparameters as part of creating the dataset.
We create highlights.jsonl
as the ground truth file containing highlights of each article stored in the test file articles.jsonl
. We store both test files in an Amazon Simple Storage Service (Amazon S3) bucket. See the following code:
Run the batch transform job
When you start a batch transform job, SageMaker launches the necessary compute resources to process the data, including CPU or GPU instances depending on the selected instance type. During the batch transform job, SageMaker automatically provisions and manages the compute resources required to process the data, including instances, storage, and networking resources. When the batch transform job is complete, the compute resources are automatically cleaned up by SageMaker. This means that the instances and storage used during the job are stopped and removed, freeing up resources and minimizing cost. See the following code:
The following is one example record from the articles.jsonl
test file. Note that record in this file has an ID that matched with predict.jsonl
file records that shows a summarized record as output from the Hugging Face Text2Text model. Similarly, the ground truth file also has a matching ID for the data record. The matching ID across the test file, ground truth file, and output file allows linking input records with output records for easy interpretation of the results.
The following is the example input record provided for summarization:
The following is the predicted output with summarization:
The following is the ground truth summarization for model evaluation purposes:
Next, we use the ground truth and predicted outputs for model evaluation.
Evaluate the model using a ROUGE score¶
ROUGE, or Recall-Oriented Understudy for Gisting Evaluation, is a set of metrics and a software package used for evaluating automatic summarization and machine translation in natural language processing. The metrics compare an automatically produced summary or translation against a reference (human-produced) summary or translation or a set of references.
In the following code, we combine the predicted and original summaries by joining them on the common key id
and use this to compute the ROUGE score:
Perform real-time batch inference
Next, we show you how to run real-time batch inference on the endpoint by providing the inputs as a list. We use the same model ID and dataset as earlier, except we take a few records from the test dataset and use them to invoke a real-time endpoint.
The following code shows how to create and deploy a real-time endpoint for real-time batch inference:
Next, we prepare our input payload. For this, we use the data that we prepared earlier and extract the first 10 test inputs and append the text inputs with hyperparameters that we want to use. We provide this payload to the real-time invoke_endpoint
. The response payload is then returned as a list of responses. See the following code:
Clean up
After you have tested the endpoint, make sure you delete the SageMaker inference endpoint and delete the model to avoid incurring charges.
Conclusion
In this notebook, we performed a batch transform to showcase the Hugging Face Text2Text Generator model for summarization tasks. Batch transform is advantageous in obtaining inferences from large datasets without requiring a persistent endpoint. We linked input records with inferences to aid in result interpretation. We used the ROUGE score to compare the test data summarization with the model-generated summarization.
Additionally, we demonstrated real-time batch inference, where you can send a small batch of data to a real-time endpoint to achieve a balance between latency and throughput for scenarios like streaming input data. Real-time batch inference helps increase throughput for real-time requests.
Try out the batch transform with Text2Text Generation models in SageMaker today and let us know your feedback!
About the authors
Hemant Singh is a Machine Learning Engineer with experience in Amazon SageMaker JumpStart and Amazon SageMaker built-in algorithms. He got his masters from Courant Institute of Mathematical Sciences and B.Tech from IIT Delhi. He has experience in working on a diverse range of machine learning problems within the domain of natural language processing, computer vision, and time series analysis.
Rachna Chadha is a Principal Solutions Architect AI/ML in Strategic Accounts at AWS. Rachna is an optimist who believes that the ethical and responsible use of AI can improve society in future and bring economic and social prosperity. In her spare time, Rachna likes spending time with her family, hiking, and listening to music.
Dr. Ashish Khetan is a Senior Applied Scientist with Amazon SageMaker built-in algorithms and helps develop machine learning algorithms. He got his PhD from University of Illinois Urbana-Champaign. He is an active researcher in machine learning and statistical inference, and has published many papers in NeurIPS, ICML, ICLR, JMLR, ACL, and EMNLP conferences.
Author: Hemant Singh